Background
Artificial intelligence (AI)-based risk stratification systems in upper gastrointestinal bleeding (UGIB) outperform existing risk scores, but successful implementation of such systems into practice requires acceptance and trust of the technology by clinicians. In this randomized trial performed in an emergency room simulation setting, we sought to assess factors impacting technology acceptance and use and to determine if a large language model (LLM) providing a human-like interface could improve acceptance, improving integration into practice.
Methods
We developed GutGPT, a LLM enhanced AI clinical decision support system (AI-CDSS) designed to better communicate and explain output from an AI risk stratification system for UGIB and provide clinical management recommendations based on UGIB guidelines. Medical students, internal medicine residents, and emergency medicine residents participated in UGIB simulation scenarios (Figure 1). Tasks during the simulation included risk stratification (at varying levels of acuity) and medical management of UGIB. Participants were randomized to receive only output from the AI risk stratification system via an interactive dashboard versus use of GutGPT along with the AI risk stratification system dashboard. The primary outcome was technology acceptance, measured across domains of Trust, Behavioral Intention, Social Influence, Facilitating Conditions, Effort Expectancy, and Performance Expectancy using a validated Unified Theory of Acceptance and Use of Technology (UTAUT) survey instrument. We also conducted a mixed methods analysis through semi-structured interviews with thematic analysis. Finally, participants received a content mastery assessment of guideline-driven UGIB management.
Results
Interim results from 55 participants (Figure 2a) suggested exposure to either GutGPT or the AI interactive dashboard maintained positive perceptions of Trust, Behavioral Intention, and Social Influence, and Performance Expectancy. (Figure 2b). Following simulations, participants in both arms reported improvement in negative emotional reactions to AI-CDSS tools and had improvement in content mastery. Themes elicited during the interview for GutGPT usability/trust include familiarity of chatbot interface, difficulty with coming up with prompts, importance of citations, importance of electronic medical record integration, and how the chatbot impacted the decision-making process of the care team.
Conclusion
Our work establishes the feasibility of studying human-algorithmic trust through simulation and of eliciting themes for further improvement in integrating LLM-enhanced AI systems into clinical care. Our initial findings suggest use of an AI-CDSS with or without LLMs in a simulation setting may improve measures of technology acceptance and use.
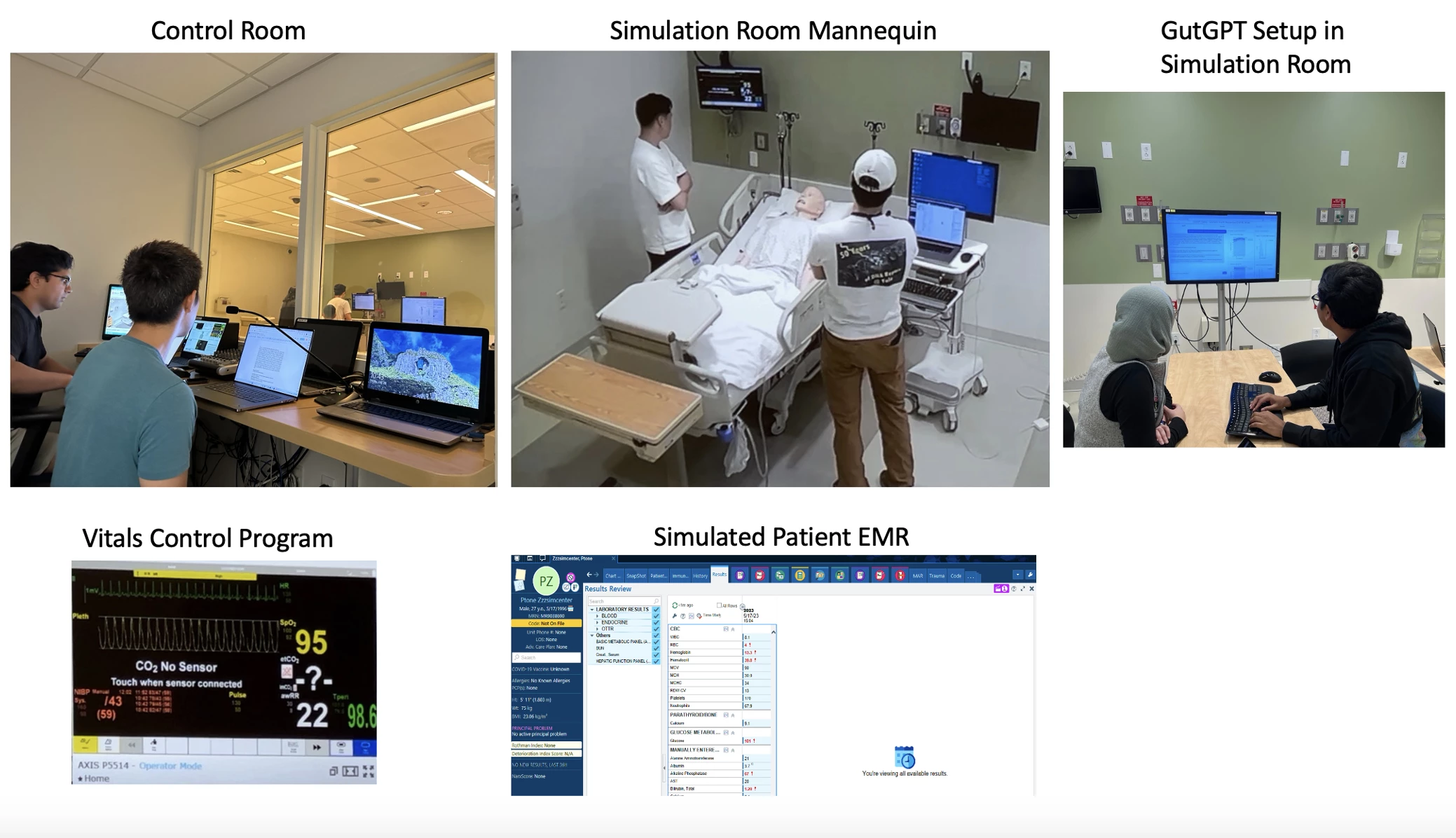
Photoset depicting typical simulation setup. The simulation is set up in the control room, which controls the mannequin and can display vitals through the control program. Simulation proctors interact with participants through the microphone. The Simulation Room contains the mannequin as well as a vitals monitor that outputs the programmed vitals. A computer workstation contains a simulated electronic medical record (EMR) that displays the patient’s data. A separate workstation in the simulation room is loaded with the GutGPT program; participants can interact with the tool as they are discussing the case and making management plans
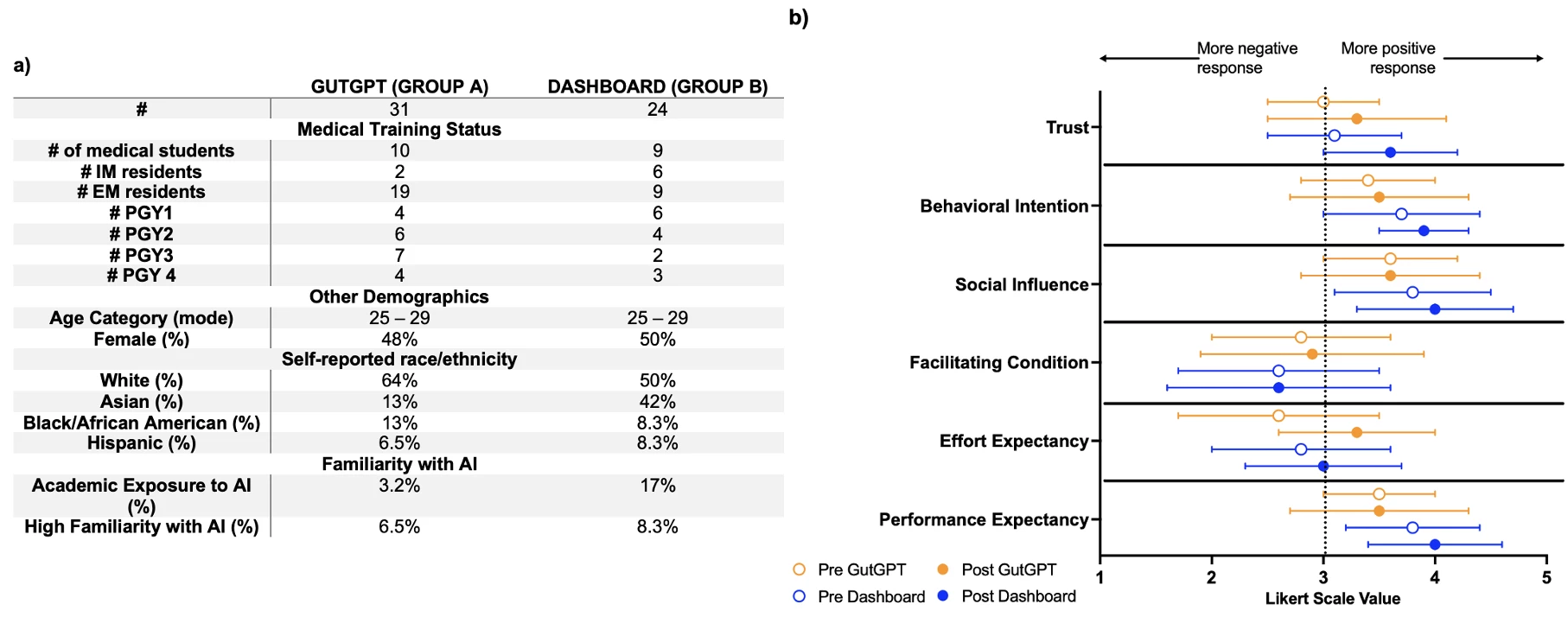
a) Demographics of participants in the trial. Note that the totality of the self-reported ethnicity/race may not equal 100% given possibility of multiple reported races/ethnicities and the inclusion of “Prefer Not to Disclose” item. b) Overall changes to UTAUT based measurements before (“Pre) and after (“Post”) simulation, stratified by the two arms in the trial design (“Dashboard” vs “GutGPT”). Results suggest overall improvement in almost all measures for both arms.