Background
Large language models (LLMs) can potentially optimize the delivery of relevant information from clinical guidelines to healthcare providers, enhancing guideline adherence and decision-making. Despite the advancements in screening and management of the Hepatitis C Virus (HCV) chronic infection, adherence to guidelines for screening and management of chronic remains challenging. This study presents an LLM pipeline that references current HCV guidelines to provide accurate point-of-care responses to questions related to screening and management of chronic HCV.
Methods
We constructed a test set of 15 questions representing each major section of the HCV guidelines. The LLM pipeline was developed in five stages: in-context learning, structured text reformatting, conversion of tables into lists, prompt engineering, and few-shot learning. We tested the LLM pipeline by generating responses to the 15 questions with 5 separate iterations by sequentially introducing each stage of the pipeline. The primary outcome was the proportion of accurate responses. We defined accuracy for each response as 1 for completely accurate or 0 otherwise. An expert hepatologist manually performed a blind grading of each response for accuracy based on the EASL guidelines. We tested each stage of the pipeline and compared the performance to the baseline GPT-4 Turbo using the Chi-Square Test. In Stage 1, we provided the pdf of the guidelines for in-context learning; in Stage 2, we provided the guidelines pdf converted into a txt file, cleaned with the removal of non-informative data (page header, references) and tables converted into csv files; in Stage 3, the cleaned text was restructured adding standardized structured wording that preceded each title, evidence and recommendations and tables were converted into lists that were integrated into the text; in Stage 4, we added prompt-engineering with instructions on format interpretation; in Stage, 5, we added few-shot learning using 54 question-answer pairs.
Results
Stage 1, incorporating in-context guidelines, improved accuracy to 72% from 50.7% (p=0.01) (Figure 1). Stage 2, incorporating cleaned in-context guidelines and converted tables to csv files, improved accuracy improved to 80% (p<0.001). Stage 3, incorporating structured guidelines and tables converted into lists, improved accuracy to 92% (p<0.001). Stage 4, adding custom prompt engineering, and Stage 5, adding few-shot learning, led to the same accuracy increase to 98.7% (p<0.001).
Conclusion
We present an LLM pipeline that produces accurate responses for guideline recommendations in chronic HCV management. Our findings suggest that LLMs could provide accurate, guideline-recommended responses with proper safeguards. Future research should establish best practices for LLM use in clinical settings.
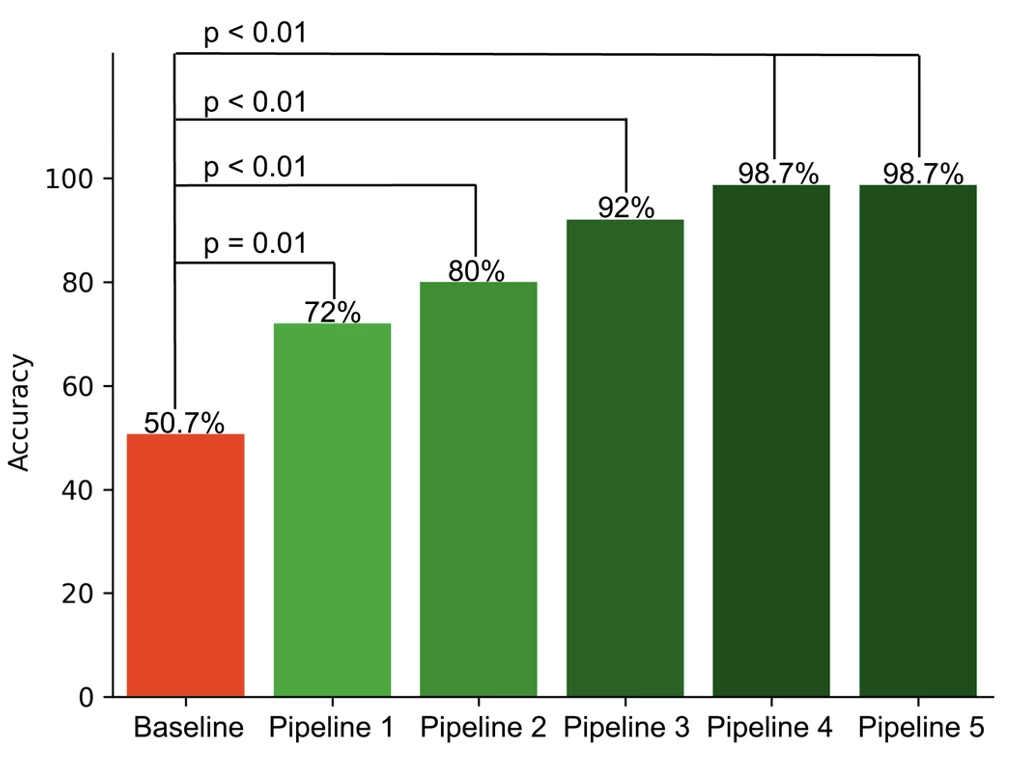
Figure 1: Qualitative evaluation of accuracy among all experiments from baseline to Pipeline 5, with increasing levels of complexity regarding prompt engineering and guidelines formatting. Accuracy is defined as the ratio between the number of completely accurate answers to the number of total answers for each experimental setting.